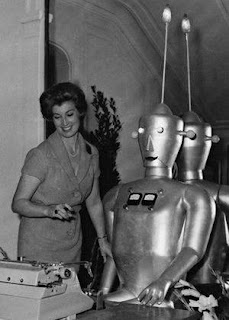
I
wrote last June about replacing traditional multi-step campaigns with a system that tracks customers through journey stages and executes short sequences of actions, called “plays”, at each stage. The goal was to approach the perfect campaign design of “do the right thing, wait, and do the right thing again”.
I still think approach makes sense but it suffers from a major flaw: someone has to define all those stages and all those plays. This limits the number of actions available since it takes considerable human time, effort, and insight to create each stage and play. Ideally, you’d let machines evolve the stages and plays autonomously. But while it’s easy for conventional predictive models to find the “next best action” in a particular situation, it’s much harder to find the best sequence of actions. This is why you can find dozens of automated products to personalize Web sites but none to automate multi-step campaign design.
The problem with multi-step campaigns is the number of options to test. These have to cover all possible actions in all possible sequences at all possible time intervals. Few companies have enough customer activity to test all the possibilities, and, even if they did, it would take unacceptably long to stumble upon the best combinations and cost unacceptable amounts of lost revenue from customers in losing test cells. In any case, available actions and customer behaviors are constantly changing, so the best approach will change over time – meaning the system would need to be constantly retesting, with all the resulting costs.
I’ve recently begun to imagine what I think is a solution. Let’s start with the problem that’s already solved, of finding the single next best action. You can conceive of my perfect campaign as a collection of next best actions. But a solution that merely executed the next best action for each customer every day would almost surely produce too many messages. One solution is a model that predicts the value* of each potential action, rather than simply ranking the actions against each other. This lets you set a minimum value for outbound messages. On days when no action meets this threshold, the system would simply do nothing. A still better approach is to explicitly consider “no action” as an option to test and build a model that gives it a value – presumably, the value being higher response to future promotions. Now you have a system that is organically evolving the timing of multi-step campaigns – and, better still, adapting that timing to behavior of each individual.
But what about sequences of related actions (i.e.,“plays”)? Let’s assume for a moment that the action sequences already exist, even if humans had to create them. This turns out to be a non-issue: if the best action to take with a customer is the second step in a sequence, the system should find that and choose it. If some other action would be more productive, we’d want to system to pick that anyway. The only caveat is the predictions must take into account previous actions, so any benefit from being part of a sequence is properly reflected in the value calculations. But a good model should consider previous actions anyway, whether or not they’re part of a formal sequence. At most, marketers might want to stop customers from receiving messages out of order. This is easy enough to design – it just becomes part of the eligibility rules that limit the actions available for a given customer. Such rules must exist for any number of reasons, such as location, products owned, or credit limit, so adding sequence constraints is little additional work. In practice, the optimal sequence for different customers is likely to be different, so imposing a fixed sequence is often actively harmful.
So far so good, but we haven’t really solved the problem of too many combinations. This requires another level of abstraction to reduce the number of options that need to be tested. When it comes to timing, initial tests of waiting for random intervals between actions should pretty quickly uncover when it’s too soon for any new action and when it’s too long to wait. This can be abstracted from results across all actions, so the learning should come quite quickly. Once reliable estimates are available, they can be used in prediction models for all possible actions. Future tests can be then limited to refining the timing within the standard range, with only a few tests outside the range to make sure nothing has changed.
The same approach could reduce other types of testing to keep the number of combinations within reason. For example, actions can be classified by broad types (cross sell, upsell, retention, winback, price level, product line, customer support, education, etc.) to quickly understand which types of actions are most productive in given situations. Testing can then focus on the top-ranked alternatives. This is relatively straightforward once actions are properly tagged with such attributes – or machine learning discovers the relevant attributes without tagging. Again, the system will still test some occasional outliers to find any new patterns that might appear.
Incidentally, this approach also helps to solve the problem of sequence creation. Category-level predictions would show when a customer is likely to respond to another action within a given category. If the system is consistently running out of fresh actions in one category, that’s a strong hint that more should be created. Thus, a sequence (or play) is born.
So – we’ve found a way for machines to design multi-step sequences and to reduce testing to a reasonable number of combinations. You might be thinking this is interesting but impractical because it requires running hundreds or thousands of models against each customer every day or possibly more often. But it turns out that’s not necessary. If we return to our concept of a value threshold and assume that time plays a role in every model score, then it’s possible to calculate in advance when the value of each action for each customer will reach the threshold. The system can then find whichever action will reach the threshold first and schedule that action to execute at that time. No further calculations are needed unless the model changes or you get new information about the customer – most likely because they did something. Of course, you’d want to recalculate the scores at that time anyway. In most businesses, such changes happen relatively rarely, so the number of customers with recalculated scores on any given day is a tiny fraction of the full base.
None of the concepts I’ve introduced here – value thresholds, explicitly testing “no action”, sharing attributes across models, and precalculating future model scores – is especially advanced. I’d be shocked if many developers hadn’t already started to use them. But I’ve yet to see a vendor pull them together into a single product or even hint they were moving in this direction. So this post is my little holiday gift – and challenge – to you, Martech Industry: it’s time to use these ideas, or better ones of your own, to reinvent campaign management as an automated system that lets marketers focus again on customers, not technology.
_______________________________________________________________________
* Value calculation is its own challenge. But marketers need to pick some value measure no matter how they build their campaigns, so lack of a perfect value measure isn't a reason to reject the rest of this argument.