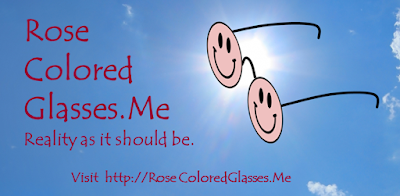
The presentation has been quite popular – especially the part about the donut. So far the world is tracking my predictions quite closely. To take one example, the script says that wireless connections to automobiles were banned after "the Minneapolis Incident of 2018". Details aren’t specified but presumably the Incident was a cyberattack that took over cars remotely. Subsequent reports of remote Jeep hacking hacking fit the scenario almost exactly and the recent take-down of the DYN DNS server by a botnet of nanny cams and smart printers was an even more prominent illustration of the danger. The resulting, and long overdue, concern about security on Internet of Things devices is just what I predicted from Minneapolis Incident.
Fond as I am of that scenario, enough has happened to justify a new one. Two particular milestones were last summer’s mass adoption of augmented reality in the form of Pokémon Go and this autumn’s sudden awareness of reality bubbles created by social media and fake news.
The new scenario describes another woman, Sue, walking down Michigan Avenue in Chicago. She’s wearing augmented reality equipment – let’s say from RoseColoredGlasses.Me, a real Web site* – that presents shows her preferred reality: one with trash removed from the street and weather changed from cloudy to sunshine. She’s also receiving her preferred stream of news (the stock market is up and the Cubs won third straight World Series). Now she gets a message that her husband just sent flowers to her office. She checks her hair in the virtual mirror – she looks marvelous, as always – and walks into a store to find her favorite brand of shoes are on sale. Et cetera.
There’s a lot going on here. We have visual alterations (invisible trash and shining sun), facts that may or may not be true (stock market and baseball scores), events with uncertain causes (did her husband send those flowers or did his computer agent?), possible self-delusion (her hair might not look so great), and commercial machinations (is that really a sale price for those shoes?). It's complicated but the net result is that Sue lives in a much nicer world than the real one. Many people would gladly pay for a similar experience. It’s the voluntary nature of this purchase that makes RoseColoredGlasses.Me nearly inevitable: there will definitely be a market. Let’s call it “personal reality”.
We have to work out some safeguards so Sue doesn’t trip over a pile of invisible trash or get run over by a truck she has chosen not to see. Those are easy to imagine. Maybe she gets BubbleBurstTM reality alerts that issue warnings when necessary. Or, less jarringly, the system might substitute things like flower beds for trash piles. Maybe the street traffic is replaced by herds of brightly colored unicorns.
If we really want things to get interesting, we can have Sue meet a friend. Is her friend experiencing the same weather, same baseball season, same unicorns? If she isn’t, how can they effectively communicate? Maybe they can switch views, perhaps as easily as trading glasses: literally seeing the world through someone else’s eyes. That could be quite a shock. Maybe Sue’s friend is the fearful type and has set her glasses to show every possible threat; not only are the trash piles highlighted but strangers look frightening and every product has a consumer warning label attached. A less disruptive approach could be some external signifier to show her friend’s current state: perhaps her glasses are tinted gray, not rose colored, or Sue sees worried-face emoticon on her forehead.
The communication problems are challenging but solvable. Still, we can expect people with similar views to gravitate towards each other. They would simply find it easier and more pleasant to interact with people sharing their views. Of course, this type of sorting happens already. That’s what makes the RoseColoredGlasses.Me scenario so intriguing: it describes highly-feasible technical developments that are entirely compatible with larger social trends and, perhaps, human nature itself. Many forces push in this direction and there’s really nothing to stop it. I have seen the futures and they work.
Maybe you’re not quite ready to give up on the notion of objective reality. If I can screen out global warming, homeless people, immigrants, Republicans, Democrats, or anything else I dislike, then what’s to motivate me to fix the actual underlying problems? Conversely, if people’s true preferences are known do they justify real-world action: say, to remove actual homeless people from the streets if no one wants to see them. That sounds ugly but maybe a market mechanism could turn it to advantage: if enough people pay RoseColoredGlasses.Me to remove the homeless people from their virtual world, then some of that money could fund programs to help the actual homeless people. Maybe that’s still immoral when people are involved but what if we’re talking about better street signs? Replacing virtual street signs for RoseColoredGlasses.Me subscribers with actual street signs visible to everyone sounds like a winner. It would even mean less work for the computers and thus save money for RoseColoredGlasses.Me.
Another wrinkle: if the owners of RoseColoredGlasses.Me are really smart (and they will be), won't they manipulate customers’ virtual reality in ways that lead the city to put up better street signs with its own money? Maybe there will be a virtual mass movement on the topic, complete with artificial-but-realistic social media posts, videos of street demonstrations, and heart-rending reports of tragic accidents that could have been avoided with better signage. Customers would have no way to know which parts were real. Then again, they can’t tell today, either.
The border between virtual and actual reality is where the really knotty problems appear. One is the fate of people who can’t afford to pay for a private reality: as we already noted, they get stuck in a world where problems don’t get solved because richer people literally don’t see them. Again, this isn’t so different from today’s world, so it may not raise any new questions (although it does make the old questions more urgent). Today’s world also hints at the likely resolution: people living in different realities will be physically segregated. Wealthier people will pay to have nicer environments and will exclude others who can’t afford the same level of service. They will avoid public spaces where different groups mix and will pay for physical and virtual buffers to manage any mixing that does occur.
Another problem is the cost of altering reality for paying customers. It’s probably cheap to insert better street signs. But masking the impact of global warming could get expensive. On a technical level, bigger changes require more processing power for the computer and better cocoons for the customers. To fix global warming they’d need something that changes the apparent temperature, precipitation, and eventually the shoreline and sea level. It’s possible to imagine RoseColoredGlasses.Me customers wearing portable shells that create artificial environments as they move about. But it's more efficient for the computer if people to stay inside and simulate the entire experience. Like most of the other things I’ve suggested here, this sounds stupid and crazy but, as anyone who has used a video conference room already knows, it’s also not so far from today’s reality. If you think I’m blurring the border between augmented and virtual reality, it’s not because I’m unaware of the distinction. It’s because the distinction is increasingly blurry.
I do think, though, that the increasing cost of having the computer generate greater deviations from physical reality will have an important impact on how things turn out. So let's pivot from discussing ever-greater personalization (the ultimate endpoint of which is personal reality) to discussing the role of computers in it all.
To start once more with the obvious, personal reality takes a lot of computer power. Beyond whatever hyperrealistic rendering is needed, the system needs vast artificial intelligence to present the reality each customer has specified. After all, the customer will only define a relatively small number of preferences, such as “there is no such thing as global warming”. It’s then up to the computer to create a plausible environment that matches that preference (to the degree possible, of course; some preferences may simply be illogical or self-contradictory). The computer also probably has to modify news feeds, historical data, research results, and other aspects of experience to match the customer’s choice.
The computer must deliver these changes as efficiently as possible – after all, RoseColoredGlasses.Me wants to make a profit. This means the computer may make choices that minimize its cost even when those choices are not in the interest of the customer. For example, if going outdoors requires hugely expensive processing to hide the actual weather, the computer might start generating realities that lead the customer to stay inside. This could be as innocent as suggesting they order in rather than visit a restaurant (especially if delivery services allow the customer to eat the same food either way). Or it could deter travel with fake news reports about bad weather, transit breakdowns, or riots. As various kinds of telepresence technology improve, keeping customers indoors will become more possible and, from the customer’s standpoint, actually a better option.
This all happens without any malevolence by the computer or its operator. It certainly doesn't matter whether the computer is self-aware. The computer is simply be optimizing results for all concerned. In practice, each personal reality involves vastly more choices than anyone can monitor, so the computer will be left to its own devices. No one will understand what the computer is doing or why. Theoretically customers could reject the service if they find the computer is making sub-optimal choices. But if the computer is controlling their entire reality, customers will have no way to know that something better is possible. Friends or news reports who tried to warn them would literally never be heard – their words would be altered to something positive. If they persisted, they would probably be blocked out entirely.
I know this all sounds horribly dystopian. It is. My problem is there’s no clear boundary between the attractive but safe applications – many of which exist today – and the more dangerous ones that could easily follow. Many people would argue that systems like Facebook have already created a primitive personal reality that is harmful to the individuals involved (and to the larger social good, if they believe that such a thing exists). So we’ve already started down the slippery slope and there’s no obvious fence to stop our fall.
Or maybe there is. It’s possible that multiple realities will prove untenable. Maybe the computers themselves will decide it’s more efficient to maintain a single reality and force everyone to accept it (but I suspect customers would rebel). Maybe social cohesion will be so damaged that a society with multiple realities cannot function (although so far that hasn’t happened). Maybe governments will decide to require degree of shared reality and limit the amount of permitted diversity (already happens in authoritarian regimes but not yet in Western democracies). Or maybe societies with a unified reality will be more effective and ultimately outcompete more fractured societies (possible and perhaps likely, but not right away). In short, the future is far from clear.
And what does all this mean for marketing? Maybe that’s a silly question when reality itself is at stake. But assuming that society doesn’t fall apart entirely, you’ll still need to make a living. Some less extreme version of what I’ve described will almost surely come to pass. Let's say it boils down to increasingly diverse personal realities as computers control larger portions of everyone’s experience. What would that imply?
One implication is the number of entities with direct access to any particular individual will decrease. Instead of dealing with Apple, Facebook, Google, and Amazon for different purposes, individuals will get a more coherent experience by selecting one gatekeeper for just about everything. This will give gatekeepers more complete information for each customer, which will let the gatekeepers drive better-tailored experiences. Marketing at gatekeepers will therefore focus on gathering as much information as possible, using it to understand customer preferences, and delivering experiences that match those preferences. Competition will be based on insights, scope of services, and efficient execution. The winners will be companies who can guide consumers to enjoy experiences that are cost-effective to deliver.
Gatekeeper marketers will still have to build trusted brands, but this will become less important. Different gatekeeping companies will probably align with different social groups or attitudes, so most people will have a natural fit with one gatekeeper or another. This social positioning will be even more important as gatekeepers provide an ever-broader range of services, making it harder to find specific points of differentiation. Diminished competition, ability to block messages from other gatekeepers, and the high cost of switching will mean customers tend to stick after their initial choice. People who do make a switch can expect great inconvenience as the new gatekeeper assembles information to provide tailored services. Switchers might even lose touch with old friends as they vanish from communication channels controlled by their former gatekeeper. In the RoseColoredGlasses.Me scenario, they could become literally invisible as they’re blocked from sight in friends' augmented realities.
Marketers who work outside the gatekeepers will face different challenges. Brand reputation and trust will again be less important since gatekeepers make most choices for consumers. In an ideal world the gatekeepers would constantly scan the market to find the best products for each customer. This would open every market to new suppliers, putting a premium on superior value and meeting customer needs. But in the real world, gatekeepers could easily get lazy. They'd offer less selection and favor suppliers who give the best deal to the gatekeeper itself. The risk is low, since customers will rarely be aware of alternatives the gatekeeper doesn’t present. New brands will pay a premium to hire the rare guerilla marketers who can circumvent the gatekeepers to reach new customers directly.
Jane in her self-driving car and Sue walking down Michigan Avenue are both headed in the same direction: they are delegating decisions to machines. But Jane is at an earlier stage in the journey, where she’s still working with different machines simultaneously – and therefore has to decide repeatedly which machines to trust. Paradoxically, Sue makes fewer choices even though she has more control over her ultimate experience. Marketers play important roles in both worlds but their tasks are slightly different. The best you can do is an eye out for signs that show where your business is now and where it’s headed. Then adjust your actions so you arrive safely at your final destination. .
_____________________________________________________________________
*The site's a joke. I no longer own the domain, though.